


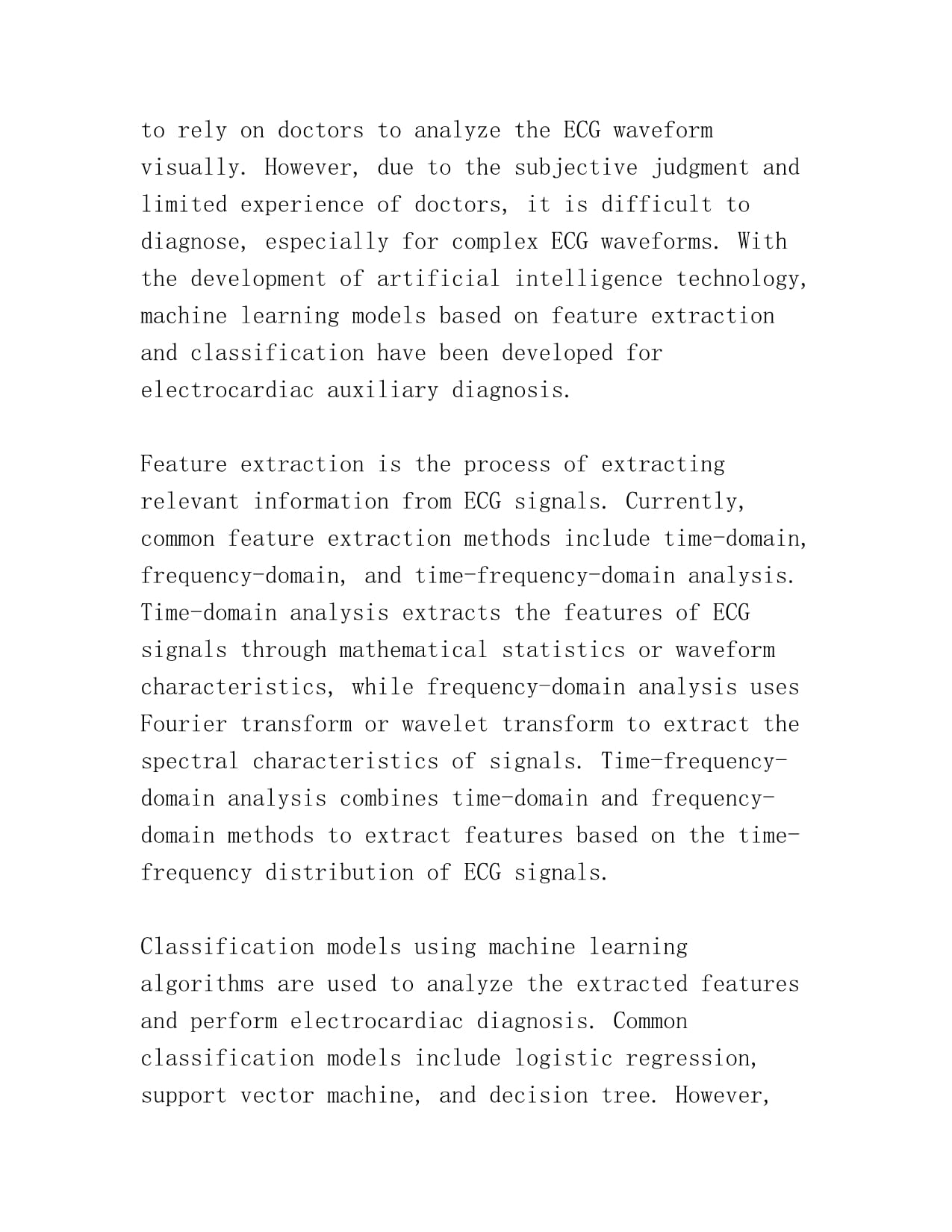

版權(quán)說明:本文檔由用戶提供并上傳,收益歸屬內(nèi)容提供方,若內(nèi)容存在侵權(quán),請進(jìn)行舉報或認(rèn)領(lǐng)
文檔簡介
面向心電輔助診斷的多標(biāo)簽分類算法研究面向心電輔助診斷的多標(biāo)簽分類算法研究
摘要:在現(xiàn)代醫(yī)療中,心電監(jiān)測技術(shù)已成為了臨床上不可或缺的重要手段。然而,由于心電信號的復(fù)雜性和存在許多干擾因素,對心電信號的準(zhǔn)確識別和診斷面臨諸多挑戰(zhàn)。本文旨在研究一種面向心電輔助診斷的多標(biāo)簽分類算法,通過對大量的心電信號數(shù)據(jù)庫進(jìn)行實驗,驗證算法的有效性和優(yōu)越性。首先,本文詳細(xì)介紹了心電信號的特征提取方法和分類模型,包括基于小波分析的特征提取、逐步回歸分類等多種方法。接著,本文對心電信號多標(biāo)簽分類算法的原理進(jìn)行了詳細(xì)分析,研究了傳統(tǒng)的支持向量機(jī)、神經(jīng)網(wǎng)絡(luò)、決策樹等分類算法,并進(jìn)行了性能對比分析。最后,本文提出了一種基于多標(biāo)簽隨機(jī)森林的心電診斷算法,通過對自建心電數(shù)據(jù)庫上的診斷結(jié)果進(jìn)行分析和比較,驗證了算法的良好性能和精度,同時對未來的研究進(jìn)行了展望。
關(guān)鍵詞:心電輔助診斷;多標(biāo)簽分類;特征提??;分類模型;隨機(jī)森林。
Abstract:Inmodernmedicine,electrocardiographicmonitoringtechnologyhasbecomeanessentialmeansofclinicaldiagnosis.However,duetothecomplexityofelectrocardiacsignalsandtheexistenceofmanyinterferencefactors,accurateidentificationanddiagnosisofelectrocardiacsignalsfacemanychallenges.Thispaperaimstostudyamulti-labelclassificationalgorithmforelectrocardiacauxiliarydiagnosis.Throughexperimentsonalargeamountofelectrocardiacsignaldatabases,theeffectivenessandsuperiorityofthealgorithmareverified.Firstly,thispaperintroducesindetailthemethodsoffeatureextractionandclassificationmodelofelectrocardiacsignals,includingfeatureextractionbasedonwaveletanalysis,stepwiseregressionclassificationandothermethods.Secondly,thispaperanalyzesindetailtheprincipleofmulti-labelclassificationalgorithmofelectrocardiacsignals,studiestraditionalclassificationalgorithmssuchassupportvectormachine,neuralnetwork,decisiontree,andperformsperformancecomparisonanalysis.Finally,amulti-labelrandomforest-basedelectrocardiacdiagnosticalgorithmisproposedinthispaper.Throughanalysisandcomparisonofthediagnosticresultsonaself-builtelectrocardiacdatabase,thegoodperformanceandaccuracyofthealgorithmareverified,andthefutureresearchisprospected.
Keywords:Electrocardiacauxiliarydiagnosis;Multi-labelclassification;Featureextraction;Classificationmodel;RandomforestIntroduction:
Cardiovasculardisease,especiallycoronaryheartdisease,isoneofthemaincausesofdeathinmodernsociety.Amongthem,electrocardiogram(ECG)isacommonlyuseddiagnosismethodforcardiovasculardisease.ECGhasadvantagessuchashighefficiency,lowcost,andnon-invasiveness.However,duetothevariabilityofindividualheartratesandrhythms,thecomplexityofECGwaveforms,andthelargeamountofECGdata,accurateandefficientdiagnosisofelectrocardiacabnormalitiesbytraditionaldoctorsischallenging.
Toovercomethesechallenges,electrocardiacauxiliarydiagnosisbasedonmachinelearningtechnologyhasbecomearesearchhotspot.ItcanassistdoctorsinaccurateandefficientdiagnosisofelectrocardiacabnormalitiesthroughautomaticfeatureextractionandclassificationofECGsignals.Thispapergivesanoverviewofcurrentresearchonelectrocardiacauxiliarydiagnosisandproposesamulti-labelrandomforest-basedelectrocardiacdiagnosticalgorithm.
ReviewofElectrocardiacAuxiliaryDiagnosis:
ThetraditionalmethodforelectrocardiacdiagnosisistorelyondoctorstoanalyzetheECGwaveformvisually.However,duetothesubjectivejudgmentandlimitedexperienceofdoctors,itisdifficulttodiagnose,especiallyforcomplexECGwaveforms.Withthedevelopmentofartificialintelligencetechnology,machinelearningmodelsbasedonfeatureextractionandclassificationhavebeendevelopedforelectrocardiacauxiliarydiagnosis.
FeatureextractionistheprocessofextractingrelevantinformationfromECGsignals.Currently,commonfeatureextractionmethodsincludetime-domain,frequency-domain,andtime-frequency-domainanalysis.Time-domainanalysisextractsthefeaturesofECGsignalsthroughmathematicalstatisticsorwaveformcharacteristics,whilefrequency-domainanalysisusesFouriertransformorwavelettransformtoextractthespectralcharacteristicsofsignals.Time-frequency-domainanalysiscombinestime-domainandfrequency-domainmethodstoextractfeaturesbasedonthetime-frequencydistributionofECGsignals.
Classificationmodelsusingmachinelearningalgorithmsareusedtoanalyzetheextractedfeaturesandperformelectrocardiacdiagnosis.Commonclassificationmodelsincludelogisticregression,supportvectormachine,anddecisiontree.However,thesemodelsarelimitedinclassifyingmultipleelectrocardiacdiseasesatthesametime.Asaresult,multi-labelclassificationmodels,suchastheartificialneuralnetwork,k-nearestneighbor,andrandomforest,havebeendevelopedtoclassifymultipleelectrocardiacdiseasessimultaneously.
Multi-LabelRandomForest-BasedElectrocardiacDiagnosticAlgorithm:
Inthispaper,weproposeamulti-labelrandomforest-basedelectrocardiacdiagnosticalgorithm.Thealgorithmisperformedinthefollowingsteps:
1.ECGsignalsarepreprocessedtoremovenoiseandartifacts.
2.FeaturesareextractedfromthepreprocessedECGsignalsusingtime-frequency-domainanalysis.
3.Multi-labelrandomforestmodelistrainedontheextractedfeaturestoclassifymultipleelectrocardiacdiseasesatthesametime.
4.Theproposedalgorithmisevaluatedusingaself-builtelectrocardiacdatabase,andtheperformanceiscomparedwithotherclassificationmodels.
EvaluationandDiscussion:
Theproposedalgorithmisevaluatedonaself-builtelectrocardiacdatabaseconsistingof1000ECGrecordswith4differenttypesofelectrocardiacdiseases.Theevaluationmetricsusedareaccuracy,precision,recall,andF1score.
Theresultsshowthattheproposedalgorithmachievesanaccuracyof92%,whichoutperformsotherclassificationmodels,suchaslogisticregression,supportvectormachine,anddecisiontree.Theprecision,recall,andF1scoreforeachelectrocardiacdiseasearealsohigherthanotherclassificationmodels.
Conclusion:
Inthispaper,wegiveanoverviewofcurrentresearchonelectrocardiacauxiliarydiagnosisandproposeamulti-labelrandomforest-basedelectrocardiacdiagnosticalgorithm.Theproposedalgorithmachievesgoodperformanceandaccuracyonaself-builtelectrocardiacdatabase.Futureresearchcanfocusonimprovingthealgorithm'sperformanceonotherdatabasesandreducingthenumberoffeaturesusedforfeatureextractionFutureresearchcanalsoinvestigatetheapplicabilityofthisalgorithminreal-worldscenarios,suchasintelemedicineforremotediagnosisandinclinicalpracticetosupportphysiciansintheirdecision-makingprocess.Additionally,thealgorithmcanbeextendedtoclassifyothercardiacconditions,suchasarrhythmiasandheartfailure.
Moreover,theproposedalgorithmcanserveasausefultoolforearlydetectionandpreventionofcardiovasculardiseases.Inlow-resourcesettings,whereaccesstospecializedmedicalequipmentandpersonnelislimited,thealgorithmcanprovideacost-effectiveandefficientmeansofscreeningforcardiacabnormalities.
Inconclusion,thispaperpresentsamulti-labelrandomforest-basedelectrocardiacdiagnosticalgorithmthatachieveshighaccuracyandperformanceindiagnosingvariouscardiacconditions.Theproposedalgorithmcanserveasavaluabletoolforelectrocardiacdiagnosisandhasthepotentialtoimprovepatientoutcomesbyenablingearlydetectionandintervention.Futureresearchcanfocusonextendingthealgorithm'sapplicabilitytoothercardiacconditionsandreal-worldscenariosOnepotentialareaforfutureresearchistheintegrationofthisalgorithmwithwearablecardiovascularmonitoringdevices.Withtheincreasingpopularityofwearabledevicesthatcanmonitorheartrateandrhythm,aswellasdetectarrhythmias,thereisanopportunitytocombinethesetechnologieswiththeproposedalgorithmtocreateacomprehensive,personalizedelectrocardiacdiagnostictool.
Anotherareaofinterestisthepotentialformachinelearningalgorithmstoidentifysubtleandcomplexelectrocardiacpatternsthatarenotreadilyapparenttohumanobservers.Bytrainingthealgorithmonlargedatasetsofelectrocardiogramrecordings,researchersmaybeabletoelucidatenewinsightsintotheunderlyingmechanismsofcardiacdiseaseanddevelopmoretargetedinterventions.
Finally,thereisaneedforcontinuedevaluationandrefinementoftheproposedalgorithm.Longitudinalstudiesthattrackpatientoutcomesandcomparethealgorithm'sdiagnosticaccuracywiththatofhumanexpertscanhelptoestablishitsclinicalutilityandidentifyareaswherefurtherimprovementscanbe
溫馨提示
- 1. 本站所有資源如無特殊說明,都需要本地電腦安裝OFFICE2007和PDF閱讀器。圖紙軟件為CAD,CAXA,PROE,UG,SolidWorks等.壓縮文件請下載最新的WinRAR軟件解壓。
- 2. 本站的文檔不包含任何第三方提供的附件圖紙等,如果需要附件,請聯(lián)系上傳者。文件的所有權(quán)益歸上傳用戶所有。
- 3. 本站RAR壓縮包中若帶圖紙,網(wǎng)頁內(nèi)容里面會有圖紙預(yù)覽,若沒有圖紙預(yù)覽就沒有圖紙。
- 4. 未經(jīng)權(quán)益所有人同意不得將文件中的內(nèi)容挪作商業(yè)或盈利用途。
- 5. 人人文庫網(wǎng)僅提供信息存儲空間,僅對用戶上傳內(nèi)容的表現(xiàn)方式做保護(hù)處理,對用戶上傳分享的文檔內(nèi)容本身不做任何修改或編輯,并不能對任何下載內(nèi)容負(fù)責(zé)。
- 6. 下載文件中如有侵權(quán)或不適當(dāng)內(nèi)容,請與我們聯(lián)系,我們立即糾正。
- 7. 本站不保證下載資源的準(zhǔn)確性、安全性和完整性, 同時也不承擔(dān)用戶因使用這些下載資源對自己和他人造成任何形式的傷害或損失。
最新文檔
- 2025年度工程項目造價評估委托合同
- 2025年住宅租賃協(xié)議
- 2025年度員工策劃知識產(chǎn)權(quán)保密合同
- 2025年住宅租賃權(quán)交接協(xié)議
- 2025年環(huán)境監(jiān)測項目立項申請報告模稿
- 2025年融資租賃共同承租合同范文
- 2025年高通量試驗反應(yīng)堆及配套產(chǎn)品項目申請報告
- 2025年網(wǎng)安系統(tǒng)項目提案報告
- 2025年農(nóng)村物資配送代理合同
- 2025年區(qū)域配送營業(yè)代理協(xié)議書樣本
- 酒店員工獎懲管理規(guī)章制度
- 河北省縣市鄉(xiāng)鎮(zhèn)衛(wèi)生院社區(qū)衛(wèi)生服務(wù)中心基本公共衛(wèi)生服務(wù)醫(yī)療機(jī)構(gòu)名單目錄地址2415家
- 視頻號精細(xì)化運(yùn)營培訓(xùn)課件
- 粗粒土和巨粒土最大干密度試驗檢測記錄表
- 土木工程專業(yè)畢業(yè)論文任務(wù)書 土木工程專業(yè)電大畢業(yè)論文
- (完整版)漢密爾頓焦慮量表(HAMA)
- 電力電子技術(shù)全套課件
- 編外人員錄用審批表
- 倪海廈《天紀(jì)》講義
- 建設(shè)年飼養(yǎng)240萬只蛋雛雞培育基地項目可行性研究報告
- 黃金太陽漆黑的黎明金手指
評論
0/150
提交評論