

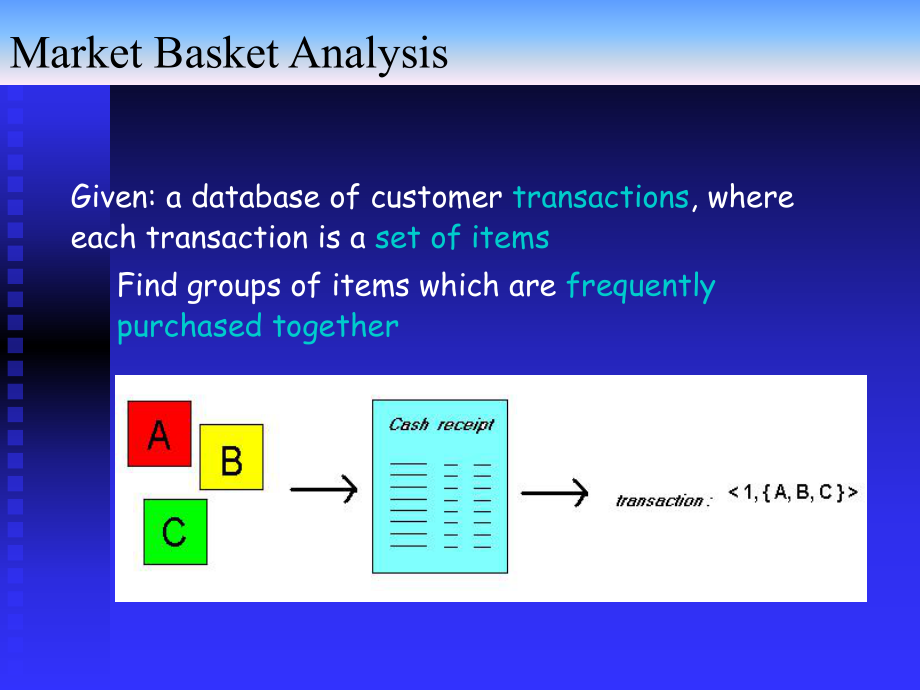


版權(quán)說明:本文檔由用戶提供并上傳,收益歸屬內(nèi)容提供方,若內(nèi)容存在侵權(quán),請進(jìn)行舉報或認(rèn)領(lǐng)
文檔簡介
1、 Customer buying habits by finding associations and correlations between the different items that customers place in their “shopping basket” Customer1Customer2Customer3Milk, eggs, sugar, breadMilk, eggs, cereal, bread Eggs, sugarMarket Basket Analysis (MBA)Given: a database of customer transactions,
2、 where each transaction is a set of itemsFind groups of items which are frequently purchased together Market Basket AnalysisMBA applicable whenever a customer purchases multiple things in proximity Goal of MBAAssociation RulesTransactions:Relational formatCompact formatItem: single element, Itemset:
3、 set of itemsSupport of an itemset I denoted by sup(I): card(I)Threshold for minimum support: Itemset I is Frequent if: sup(I) .Frequent Itemset represents set of items which arepositively correlatedBasic Concepts itemset sup(dairy) = 3 sup(fruit) = 3 sup(dairy, fruit) = 2 If = 3, then dairy and fru
4、it are frequent while dairy,fruit is not. Customer 1 Customer 2Frequent ItemsetsTransaction IDItems Bought1dairy,fruit2dairy,fruit, vegetable3dairy4fruit, cerealsq A,B - partition of a set of items q r = A B Support of r: sup(r) = sup(AB)Confidence of r: conf(r) = sup(AB)/sup(A)q Thresholds:u minimu
5、m support - su minimum confidence cr AS(s, c), if sup(r) s and conf(r) cAssociation Rules: AR(s,c)Transaction IDItems Bought2000A,B,C1000A,C4000A,D5000B,E,FFrequent Itemset SupportA75%B50%C50%A,C50%Min. support 2 50%Min. confidence - 50%Association Rules - ExampleThe Apriori algorithm Agrawalabcdc,
6、db, db, ca, da, ca, ba, b, db, c, da, c, da, b, ca,b,c,da,d is not frequent, so the 3-itemsets a,b,d, a,c,d and the 4-itemset a,b,c,d, are not generated.Apriori - ExampleAlgorithm Apriori: IllustrationuMining association rules is composed of two steps:TID Items1000 A, B, C2000 A, C3000 A, D4000 B, E
7、, F1. discover the large items, i.e., the sets of itemsets that have transaction support above a predetermined minimum support s.2. Use the large itemsets to generate the association rules A 3 B 2C 2A,C 2 Large support itemsMinSup = 2TID Items100 A, C, D200 B, C, E300 A, B, C, E400 B, E Database DA
8、B C D E Itemset CountA 2 B 3C 3E 3Itemset CountA, B A, C A, E B, C B, EC, E Itemset A,B A,C A,E B,C B,E C,E Itemset Count A, C 2 B, C 2 B, E 3C, E 2 Itemset Count B, C, E Itemset B, C, E 2 Itemset Count B, C, E 2 Itemset Count C1F1C2F2C2C3F3C3ScanDScanDScanDS = 22 3 3 1 3 1 2 1 2 3 2 Representative
9、Association RulesTransactions:A,B,C,D,EA,B,C,D,E,FA,B,C,D,E,H,IA,B,EB,C,D,E,H,IRepresentative Association RulesFind RR(2,80%)Representative Rules From (BCDEHI): H B,C,D,E,I I B,C,D,E,HFrom (ABCDE):A,C B,D,EA,D B,C,ETransactions:abcdeabcacdebcdebcbdecdeFrequent Pattern (FP) Growth StrategyMinimum Sup
10、port = 2Frequent Items:c 6b 5d 5e 5a 3Transactionsordered:cbdeacbacdeacbdecbbdecdeFP-treeFrequent Pattern (FP) Growth StrategyMining the FP-tree for frequent itemsets:Start from each item and construct a subdatabase of transactions (prefix paths) with that item listed at the end. Reorder the prefix
11、paths in support descending order. Build a conditional FP-tree.a 3 Prefix path:(c b d e a, 1)(c b a, 1)(c d e a, 1)Correct order:c 3b 2d 2e 2Frequent Pattern (FP) Growth Strategya 3 Prefix path:(c b d e a, 1)(c b a, 1)(c d e a, 1)Frequent Itemsets:(c a, 3)(c b a, 2)(c d a, 2)(c d e a, 2)(c e a, 2)Mu
12、ltidimensional ARAssociations between values of different attributes :RULES:nationality = French income = high 50%, 100%income = high nationality = French 50%, 75%age = 50 nationality = Italian 33%, 100%Multi-dimensional Single-dimensional Schema: Single-dimensional AR vs Multi-dimensionalQuantitati
13、ve AttributesProblem: too many distinct valuesSolution: transform quantitative attributes into categorical ones via discretization. Discretization of quantitative attributesConstraint-based ARApriori property revisitedMining Association Rules with ConstraintsMultilevel ARProductFam ilySectorDepartm
14、entF ro z e nR e frig e ra te dV e g e ta b leB a n a n a A p p le O ra n g e E tc .F ru itD a iryE tc .F re s hB a k e ryE tc .F o o d S tu ffHierarchy of conceptsFreshsupport = 20%Dairy support = 6%Fruit support = 1%Vegetable support = 7%q Support and Confidence of Multilevel Association RulesHier
15、archical attributes: age, salaryAssociation Rule: (age, young) (salary, 40k) ageyoung middle-aged old salarylow medium high 18 29 30 60 61 8010k40k 50k 60k 70k 80k100kCandidate Association Rules: (age, 18 ) (salary, 40k), (age, young) (salary, low), (age, 18 ) (salary, low)Mining Multilevel ARMining Multilevel ARMulti-level Assoc
溫馨提示
- 1. 本站所有資源如無特殊說明,都需要本地電腦安裝OFFICE2007和PDF閱讀器。圖紙軟件為CAD,CAXA,PROE,UG,SolidWorks等.壓縮文件請下載最新的WinRAR軟件解壓。
- 2. 本站的文檔不包含任何第三方提供的附件圖紙等,如果需要附件,請聯(lián)系上傳者。文件的所有權(quán)益歸上傳用戶所有。
- 3. 本站RAR壓縮包中若帶圖紙,網(wǎng)頁內(nèi)容里面會有圖紙預(yù)覽,若沒有圖紙預(yù)覽就沒有圖紙。
- 4. 未經(jīng)權(quán)益所有人同意不得將文件中的內(nèi)容挪作商業(yè)或盈利用途。
- 5. 人人文庫網(wǎng)僅提供信息存儲空間,僅對用戶上傳內(nèi)容的表現(xiàn)方式做保護(hù)處理,對用戶上傳分享的文檔內(nèi)容本身不做任何修改或編輯,并不能對任何下載內(nèi)容負(fù)責(zé)。
- 6. 下載文件中如有侵權(quán)或不適當(dāng)內(nèi)容,請與我們聯(lián)系,我們立即糾正。
- 7. 本站不保證下載資源的準(zhǔn)確性、安全性和完整性, 同時也不承擔(dān)用戶因使用這些下載資源對自己和他人造成任何形式的傷害或損失。
最新文檔
- 2025年度蔬菜冷鏈倉儲與配送服務(wù)合同3篇
- 2025年全球及中國常規(guī)印制電路板行業(yè)頭部企業(yè)市場占有率及排名調(diào)研報告
- 2025-2030全球協(xié)作機(jī)器人氣動夾爪行業(yè)調(diào)研及趨勢分析報告
- 2025-2030全球無線電干擾抑制濾波器行業(yè)調(diào)研及趨勢分析報告
- 專業(yè)鋼琴購銷協(xié)議示范文本2024版B版
- 專業(yè)室內(nèi)設(shè)計師2024年服務(wù)協(xié)議樣本版B版
- 2025年全球及中國含銀藻酸鹽敷料行業(yè)頭部企業(yè)市場占有率及排名調(diào)研報告
- 2025年技術(shù)轉(zhuǎn)讓付款協(xié)議2篇
- 2025年度餐廳食材供應(yīng)承包協(xié)議3篇
- 二零二五版體育場館場地租賃與賽事組織合同3篇
- 人教版(2024)英語七年級上冊單詞表
- 中醫(yī)養(yǎng)生產(chǎn)業(yè)現(xiàn)狀及發(fā)展趨勢分析
- 2023年浙江省溫州市中考數(shù)學(xué)真題含解析
- 窗簾采購?fù)稑?biāo)方案(技術(shù)方案)
- 司庫體系建設(shè)
- 居間合同范本解
- 機(jī)電傳動單向數(shù)控平臺-礦大-機(jī)械電子-有圖
- 婦科病盆腔炎病例討論
- 人教版高中物理必修一同步課時作業(yè)(全冊)
- 食堂油鍋起火演練方案及流程
- 有余數(shù)的除法算式300題
評論
0/150
提交評論